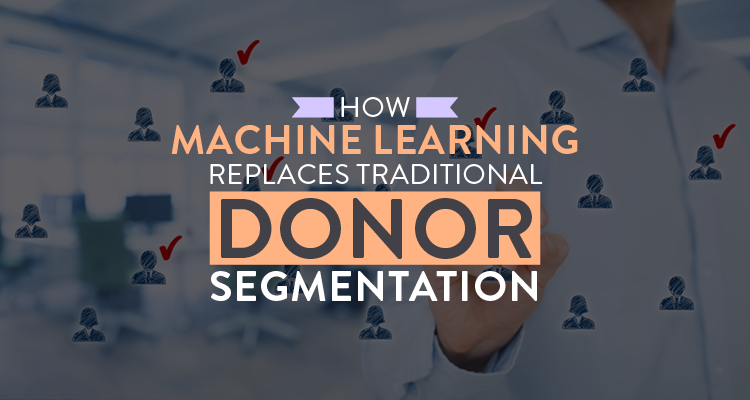
We’re entering a whole new era of fundraising. The crises of the past year, although very challenging, have also opened up new opportunities for nonprofits to strengthen their strategies, especially when it comes to technology.
Many organizations have invested in new tools to help them fundraise in smarter ways online over the past year. But top-level, donor-facing tools like fundraising and event software don’t necessarily help you improve your strategies at a deeper level.
To truly strengthen your fundraising approach from the ground up, you have to start at the root of the fundraising process—donor data—and find ways to improve (or, we argue, completely overhaul) how you use it to inform your campaigns and appeals.
Artificial intelligence for nonprofits is a new tool that can help organizations raise money more efficiently by strengthening their fundraising strategies at a foundational level. Specifically, it can replace the traditional, labor-intensive, and often inaccurate donor segmentation processes that nonprofits have historically used. Let’s explore how.
How Traditional Segmentation Falls Flat
First, let’s review the fundamentals:
What is donor segmentation?
Donor segmentation is the process of sorting your donors into distinct groups based on what you know about them—their giving habits, lifetime value, location, age, etc.
By sorting them this way, you can target your appeals and messages to the specific audiences who’ll be most likely to engage with them, therefore boosting their effectiveness.
In everyday fundraising contexts, nonprofits often rely on RFM segmentation, which takes into account your donors’ giving Recency, Frequency, and Monetary value. This gives you a general idea of who is (and isn’t) engaged and how much they give on average. It’s the most common form of donor segmentation, and your nonprofit likely already uses it in one way or another when developing appeals and other campaigns. However, it has its drawbacks.
What are the drawbacks of traditional segmentation?
Although an historically foundational part of nonprofit fundraising and communication, traditional segmentation has some serious limitations:
- It’s time-consuming. Even with a robust database to store your fundraising data, traditional segmentation still requires you to start by manually sorting and filtering your donors. This eats up your team’s time that could otherwise go towards fundraising and building donor relationships.
- It’s often vague and inaccurate. The purpose of segmentation is to help you better target specific audiences, but grouping them into clusters based on past actions usually doesn’t go far enough. A lot of valuable insights (and potential donations) can fall through the cracks when you only take a handful of data points into account.
- It’s reactive rather than proactive. Segmentation helps you make loose assumptions about how donors will act based on how they’ve acted in the past. This can be helpful, but it’s not proactive. A more modern approach can help you predict donor behaviors in advance so that you can secure more gifts, prevent donor churn, and identify growth opportunities early.
The bottom line is that traditional segmentation strategies often aren’t as efficient or effective as they could be. Nonprofits have tight budgets and calendars. If there are smarter, faster ways to segment your audience and raise more money for your mission, you should explore them!
Why Machine Learning Can Replace Segmentation
At Dataro, we believe that machine learning is the best segmentation. Consider this real-life example:
In 10 real fundraising campaigns, we compared donor response rates from mailing lists generated by our AI tools to those from the top segments from lists generated through traditional RFM analysis. Dataro’s mailing lists generated much higher response rates, resulting in more conversions and more dollars raised with less effort.
Here are the reasons why machine learning outperformed RFM segmentation:
- Machine learning works through hard data, not assumptions.
- Machine learning works by analyzing complex patterns in all of your nonprofit’s historical data, not just a handful of broad RFM metrics. This is what allows AI software to make accurate predictions down to the individual level. Without this approach, you’d instead have to rely on vague groupings of donors that don’t take all the possible and relevant factors into account—meaning missed donation opportunities and more of your team’s time wasted.
- Machine learning lets you predict future behaviors at an individual level.
- With a traditional approach, you have to guess who’ll be receptive to an appeal based on how they’ve given in the past. In contrast, machine learning generates actual predictions based on complex patterns in your data. With AI, you can proactively determine who’s ready to give and build more targeted lists from the start. These individualized predictions are invaluable for more efficient fundraising and for strengthening your relationships with donors.
- Machine learning saves you time and money.
- Machine learning can significantly boost the ROI of your campaigns. By understanding exactly who you should be targeting and when, you can better allocate your resources and boost results. Here’s a simple example: Printing direct mail appeals is expensive. With AI pointing you to the individual donors who are likely to give right now, you can immediately reduce costs by sending less wasted mail and increase your conversion rates.
Modern multi-channel communication and fundraising can be complicated and costly. But with data-driven predictions generated through machine learning, nonprofits can change how they fundraise—no more time-consuming data analysis or inaccurate mailing lists that cost more than they bring in.
Plus, AI can predict more than just a donor’s likelihood to give to a campaign. It can also proactively show you donors who are at risk of lapsing out of your regular giving program, ready to upgrade their recurring donation, or even likely to make a mid-level or major gift. The money saved through reduced churn and generated by strengthened stewardship makes machine learning an incredible asset for your mission.
Examples of Machine Learning in Action
Here are two examples of how machine learning delivers more value than traditional segmentation strategies:
Example 1: A donor has lapsed out of your regular giving program, but they’ve continued to engage with your nonprofit in other ways, like attending events. Now, you’re planning a new direct mail appeal and putting together a mailing list.
If you use traditional RFM segmentation to build your list, this lapsed donor would probably be excluded because they haven’t given a donation recently. Instead, they may just need a nudge to start giving again. Machine learning takes all of their touchpoints into account (not just donations) by studying your entire database of fundraising and engagement analytics. AI could instead flag this donor as likely to give to your appeal, making it easy to include them, secure a donation, and re-engage them for future gifts.
Example 2: A donor only gives at specific times of the year, like to your year-end fundraising campaign. You’re planning a new fundraising campaign and event for June and need to put together a list for your appeals and invites.
Since this donor technically gave within the past 12 months, RFM segmentation would consider them to be generally active—but you need a more granular understanding. In reality, this donor probably wouldn’t give to your June campaign. AI-driven predictive scores would show you that this donor is unlikely to engage with your new campaign, saving you time and money by not reaching out to them while also helping you learn more about their giving habits as an individual.
The benefits of using machine learning to proactively identify donors for appeals and outreach can’t be understated.
Especially considering the drawbacks of traditional donor segmentation (many of which organizations might not even be aware of), exploring your AI options is a worthwhile exercise. This technology is more accessible for nonprofits than ever before, and it can drastically improve your fundraising strategies at a fundamental level. Looking ahead to the rest of 2021 and beyond, machine learning could be the perfect addition to your nonprofit’s tech stack.
Author: Chris Paver, COO, Dataro
Chris is the COO of Dataro, joining the team as a business partner in 2018. Chris has a wealth of experience from the world of commercial law, where he specialized in advising technology, media, and high-growth businesses across the full spectrum of strategic, commercial, and regulatory issues. As COO, Chris has helped drive Dataro’s rapid growth in the nonprofit sector.